Pandas: groupby to sum subsets of columns
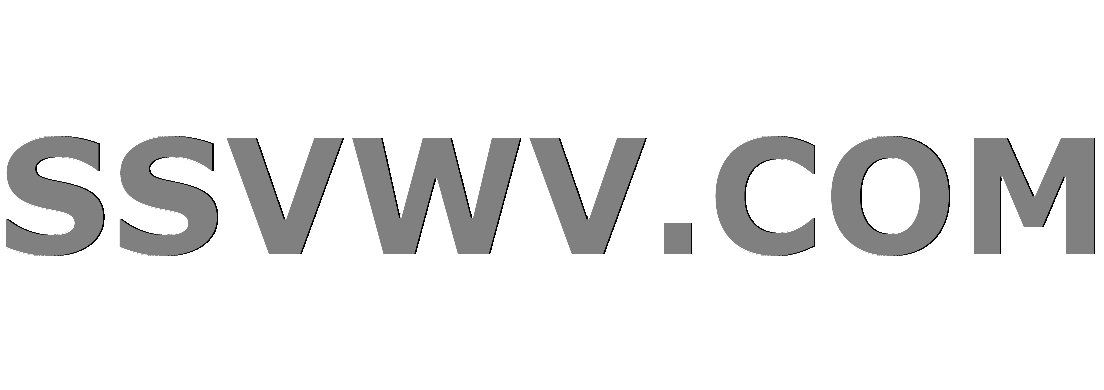
Multi tool use
up vote
0
down vote
favorite
I have a pandas dataframe with multiple columns. I would like to calculate the sum of various subsets of this columns and assign a name to each group of columns.
Is it possible to achieve this using groupby or other pandas methods?
Setup:
import numpy as np; np.random.seed(1)
import pandas as pd
df = pd.DataFrame(np.random.randint(0, 10, (3, 5)), columns=['A', 'B', 'C', 'D', 'E'])
columns_groups = {'First': ['A', 'B', 'C'],
'Second': ['D', 'E'],
'Some': ['A', 'C', 'D'],
'All': ['A', 'B', 'C', 'D', 'E']}
Desired output: (is there a more elegant solution?)
out = {}
for name, group in columns_groups.items():
out[name] = df[group].sum(axis=1)
out = pd.DataFrame(out)
out
Out[22]:
All First Second Some
0 27 22 5 19
1 23 8 15 13
2 17 11 6 9
My attempt:
df.groupby(columns_groups, axis=1).sum(axis=1)
Out[21]:
Empty DataFrame
Columns:
Index: [0, 1, 2]
python pandas
add a comment |
up vote
0
down vote
favorite
I have a pandas dataframe with multiple columns. I would like to calculate the sum of various subsets of this columns and assign a name to each group of columns.
Is it possible to achieve this using groupby or other pandas methods?
Setup:
import numpy as np; np.random.seed(1)
import pandas as pd
df = pd.DataFrame(np.random.randint(0, 10, (3, 5)), columns=['A', 'B', 'C', 'D', 'E'])
columns_groups = {'First': ['A', 'B', 'C'],
'Second': ['D', 'E'],
'Some': ['A', 'C', 'D'],
'All': ['A', 'B', 'C', 'D', 'E']}
Desired output: (is there a more elegant solution?)
out = {}
for name, group in columns_groups.items():
out[name] = df[group].sum(axis=1)
out = pd.DataFrame(out)
out
Out[22]:
All First Second Some
0 27 22 5 19
1 23 8 15 13
2 17 11 6 9
My attempt:
df.groupby(columns_groups, axis=1).sum(axis=1)
Out[21]:
Empty DataFrame
Columns:
Index: [0, 1, 2]
python pandas
P.s. suggestions for a better title are welcome
– FLab
Nov 22 at 15:40
Any reason you're specifically after agroupby
method?
– Jon Clements♦
Nov 22 at 15:49
I "feel" like this case might be covered by groupby, so I'd like to know more about its syntax or other pandas methods
– FLab
Nov 22 at 16:01
I really like the idea of using adictionary
like this togroupby
but unfortunately it is not possible afaik. If wedf.transpose()
we could technically usedf.groupby(dictionary)
to group by index and name our groups, but thenkey
has to be the value inindex
andvalue
the name of our group. This obv wont work in this case since the index will occur multiple times in different groups. Would be a cool feature though (to be able to do it on your kind ofdict
) :D
– user3471881
Nov 22 at 17:13
add a comment |
up vote
0
down vote
favorite
up vote
0
down vote
favorite
I have a pandas dataframe with multiple columns. I would like to calculate the sum of various subsets of this columns and assign a name to each group of columns.
Is it possible to achieve this using groupby or other pandas methods?
Setup:
import numpy as np; np.random.seed(1)
import pandas as pd
df = pd.DataFrame(np.random.randint(0, 10, (3, 5)), columns=['A', 'B', 'C', 'D', 'E'])
columns_groups = {'First': ['A', 'B', 'C'],
'Second': ['D', 'E'],
'Some': ['A', 'C', 'D'],
'All': ['A', 'B', 'C', 'D', 'E']}
Desired output: (is there a more elegant solution?)
out = {}
for name, group in columns_groups.items():
out[name] = df[group].sum(axis=1)
out = pd.DataFrame(out)
out
Out[22]:
All First Second Some
0 27 22 5 19
1 23 8 15 13
2 17 11 6 9
My attempt:
df.groupby(columns_groups, axis=1).sum(axis=1)
Out[21]:
Empty DataFrame
Columns:
Index: [0, 1, 2]
python pandas
I have a pandas dataframe with multiple columns. I would like to calculate the sum of various subsets of this columns and assign a name to each group of columns.
Is it possible to achieve this using groupby or other pandas methods?
Setup:
import numpy as np; np.random.seed(1)
import pandas as pd
df = pd.DataFrame(np.random.randint(0, 10, (3, 5)), columns=['A', 'B', 'C', 'D', 'E'])
columns_groups = {'First': ['A', 'B', 'C'],
'Second': ['D', 'E'],
'Some': ['A', 'C', 'D'],
'All': ['A', 'B', 'C', 'D', 'E']}
Desired output: (is there a more elegant solution?)
out = {}
for name, group in columns_groups.items():
out[name] = df[group].sum(axis=1)
out = pd.DataFrame(out)
out
Out[22]:
All First Second Some
0 27 22 5 19
1 23 8 15 13
2 17 11 6 9
My attempt:
df.groupby(columns_groups, axis=1).sum(axis=1)
Out[21]:
Empty DataFrame
Columns:
Index: [0, 1, 2]
python pandas
python pandas
edited Nov 22 at 16:01
asked Nov 22 at 15:39
FLab
3,5431941
3,5431941
P.s. suggestions for a better title are welcome
– FLab
Nov 22 at 15:40
Any reason you're specifically after agroupby
method?
– Jon Clements♦
Nov 22 at 15:49
I "feel" like this case might be covered by groupby, so I'd like to know more about its syntax or other pandas methods
– FLab
Nov 22 at 16:01
I really like the idea of using adictionary
like this togroupby
but unfortunately it is not possible afaik. If wedf.transpose()
we could technically usedf.groupby(dictionary)
to group by index and name our groups, but thenkey
has to be the value inindex
andvalue
the name of our group. This obv wont work in this case since the index will occur multiple times in different groups. Would be a cool feature though (to be able to do it on your kind ofdict
) :D
– user3471881
Nov 22 at 17:13
add a comment |
P.s. suggestions for a better title are welcome
– FLab
Nov 22 at 15:40
Any reason you're specifically after agroupby
method?
– Jon Clements♦
Nov 22 at 15:49
I "feel" like this case might be covered by groupby, so I'd like to know more about its syntax or other pandas methods
– FLab
Nov 22 at 16:01
I really like the idea of using adictionary
like this togroupby
but unfortunately it is not possible afaik. If wedf.transpose()
we could technically usedf.groupby(dictionary)
to group by index and name our groups, but thenkey
has to be the value inindex
andvalue
the name of our group. This obv wont work in this case since the index will occur multiple times in different groups. Would be a cool feature though (to be able to do it on your kind ofdict
) :D
– user3471881
Nov 22 at 17:13
P.s. suggestions for a better title are welcome
– FLab
Nov 22 at 15:40
P.s. suggestions for a better title are welcome
– FLab
Nov 22 at 15:40
Any reason you're specifically after a
groupby
method?– Jon Clements♦
Nov 22 at 15:49
Any reason you're specifically after a
groupby
method?– Jon Clements♦
Nov 22 at 15:49
I "feel" like this case might be covered by groupby, so I'd like to know more about its syntax or other pandas methods
– FLab
Nov 22 at 16:01
I "feel" like this case might be covered by groupby, so I'd like to know more about its syntax or other pandas methods
– FLab
Nov 22 at 16:01
I really like the idea of using a
dictionary
like this to groupby
but unfortunately it is not possible afaik. If we df.transpose()
we could technically use df.groupby(dictionary)
to group by index and name our groups, but then key
has to be the value in index
and value
the name of our group. This obv wont work in this case since the index will occur multiple times in different groups. Would be a cool feature though (to be able to do it on your kind of dict
) :D– user3471881
Nov 22 at 17:13
I really like the idea of using a
dictionary
like this to groupby
but unfortunately it is not possible afaik. If we df.transpose()
we could technically use df.groupby(dictionary)
to group by index and name our groups, but then key
has to be the value in index
and value
the name of our group. This obv wont work in this case since the index will occur multiple times in different groups. Would be a cool feature though (to be able to do it on your kind of dict
) :D– user3471881
Nov 22 at 17:13
add a comment |
2 Answers
2
active
oldest
votes
up vote
1
down vote
accepted
Just a different and fun way , using reindex
with MultiIndex
df=df.reindex(columns=sum(columns_groups.values(),))
t=[(x,z ) for x , y in columns_groups.items() for z in y]
df.columns=pd.MultiIndex.from_tuples(t)
df.sum(level=0,axis=1)
First Second Some All
0 22 8 18 30
1 17 9 16 26
2 6 15 14 21
1
Not planning to use it in my code, but it does indeed give a 'group-by' like solution
– FLab
Dec 5 at 17:41
add a comment |
up vote
1
down vote
Is this okay with you:
pd.DataFrame({k: df[v].sum(axis=1) for k, v in columns_groups.items()})
All First Second Some
0 27 22 5 19
1 23 8 15 13
2 17 11 6 9
It's same what you did, only in comprehension.
add a comment |
2 Answers
2
active
oldest
votes
2 Answers
2
active
oldest
votes
active
oldest
votes
active
oldest
votes
up vote
1
down vote
accepted
Just a different and fun way , using reindex
with MultiIndex
df=df.reindex(columns=sum(columns_groups.values(),))
t=[(x,z ) for x , y in columns_groups.items() for z in y]
df.columns=pd.MultiIndex.from_tuples(t)
df.sum(level=0,axis=1)
First Second Some All
0 22 8 18 30
1 17 9 16 26
2 6 15 14 21
1
Not planning to use it in my code, but it does indeed give a 'group-by' like solution
– FLab
Dec 5 at 17:41
add a comment |
up vote
1
down vote
accepted
Just a different and fun way , using reindex
with MultiIndex
df=df.reindex(columns=sum(columns_groups.values(),))
t=[(x,z ) for x , y in columns_groups.items() for z in y]
df.columns=pd.MultiIndex.from_tuples(t)
df.sum(level=0,axis=1)
First Second Some All
0 22 8 18 30
1 17 9 16 26
2 6 15 14 21
1
Not planning to use it in my code, but it does indeed give a 'group-by' like solution
– FLab
Dec 5 at 17:41
add a comment |
up vote
1
down vote
accepted
up vote
1
down vote
accepted
Just a different and fun way , using reindex
with MultiIndex
df=df.reindex(columns=sum(columns_groups.values(),))
t=[(x,z ) for x , y in columns_groups.items() for z in y]
df.columns=pd.MultiIndex.from_tuples(t)
df.sum(level=0,axis=1)
First Second Some All
0 22 8 18 30
1 17 9 16 26
2 6 15 14 21
Just a different and fun way , using reindex
with MultiIndex
df=df.reindex(columns=sum(columns_groups.values(),))
t=[(x,z ) for x , y in columns_groups.items() for z in y]
df.columns=pd.MultiIndex.from_tuples(t)
df.sum(level=0,axis=1)
First Second Some All
0 22 8 18 30
1 17 9 16 26
2 6 15 14 21
answered Nov 22 at 16:04


W-B
97.3k73162
97.3k73162
1
Not planning to use it in my code, but it does indeed give a 'group-by' like solution
– FLab
Dec 5 at 17:41
add a comment |
1
Not planning to use it in my code, but it does indeed give a 'group-by' like solution
– FLab
Dec 5 at 17:41
1
1
Not planning to use it in my code, but it does indeed give a 'group-by' like solution
– FLab
Dec 5 at 17:41
Not planning to use it in my code, but it does indeed give a 'group-by' like solution
– FLab
Dec 5 at 17:41
add a comment |
up vote
1
down vote
Is this okay with you:
pd.DataFrame({k: df[v].sum(axis=1) for k, v in columns_groups.items()})
All First Second Some
0 27 22 5 19
1 23 8 15 13
2 17 11 6 9
It's same what you did, only in comprehension.
add a comment |
up vote
1
down vote
Is this okay with you:
pd.DataFrame({k: df[v].sum(axis=1) for k, v in columns_groups.items()})
All First Second Some
0 27 22 5 19
1 23 8 15 13
2 17 11 6 9
It's same what you did, only in comprehension.
add a comment |
up vote
1
down vote
up vote
1
down vote
Is this okay with you:
pd.DataFrame({k: df[v].sum(axis=1) for k, v in columns_groups.items()})
All First Second Some
0 27 22 5 19
1 23 8 15 13
2 17 11 6 9
It's same what you did, only in comprehension.
Is this okay with you:
pd.DataFrame({k: df[v].sum(axis=1) for k, v in columns_groups.items()})
All First Second Some
0 27 22 5 19
1 23 8 15 13
2 17 11 6 9
It's same what you did, only in comprehension.
answered Nov 22 at 15:46


zipa
15.8k21435
15.8k21435
add a comment |
add a comment |
Thanks for contributing an answer to Stack Overflow!
- Please be sure to answer the question. Provide details and share your research!
But avoid …
- Asking for help, clarification, or responding to other answers.
- Making statements based on opinion; back them up with references or personal experience.
To learn more, see our tips on writing great answers.
Some of your past answers have not been well-received, and you're in danger of being blocked from answering.
Please pay close attention to the following guidance:
- Please be sure to answer the question. Provide details and share your research!
But avoid …
- Asking for help, clarification, or responding to other answers.
- Making statements based on opinion; back them up with references or personal experience.
To learn more, see our tips on writing great answers.
Sign up or log in
StackExchange.ready(function () {
StackExchange.helpers.onClickDraftSave('#login-link');
});
Sign up using Google
Sign up using Facebook
Sign up using Email and Password
Post as a guest
Required, but never shown
StackExchange.ready(
function () {
StackExchange.openid.initPostLogin('.new-post-login', 'https%3a%2f%2fstackoverflow.com%2fquestions%2f53434296%2fpandas-groupby-to-sum-subsets-of-columns%23new-answer', 'question_page');
}
);
Post as a guest
Required, but never shown
Sign up or log in
StackExchange.ready(function () {
StackExchange.helpers.onClickDraftSave('#login-link');
});
Sign up using Google
Sign up using Facebook
Sign up using Email and Password
Post as a guest
Required, but never shown
Sign up or log in
StackExchange.ready(function () {
StackExchange.helpers.onClickDraftSave('#login-link');
});
Sign up using Google
Sign up using Facebook
Sign up using Email and Password
Post as a guest
Required, but never shown
Sign up or log in
StackExchange.ready(function () {
StackExchange.helpers.onClickDraftSave('#login-link');
});
Sign up using Google
Sign up using Facebook
Sign up using Email and Password
Sign up using Google
Sign up using Facebook
Sign up using Email and Password
Post as a guest
Required, but never shown
Required, but never shown
Required, but never shown
Required, but never shown
Required, but never shown
Required, but never shown
Required, but never shown
Required, but never shown
Required, but never shown
DmmDquB8tFn2ZEbLUyg7Y9hld,VJN rXFogMSNzISBzlSrfkF3 H9zkwipFSb
P.s. suggestions for a better title are welcome
– FLab
Nov 22 at 15:40
Any reason you're specifically after a
groupby
method?– Jon Clements♦
Nov 22 at 15:49
I "feel" like this case might be covered by groupby, so I'd like to know more about its syntax or other pandas methods
– FLab
Nov 22 at 16:01
I really like the idea of using a
dictionary
like this togroupby
but unfortunately it is not possible afaik. If wedf.transpose()
we could technically usedf.groupby(dictionary)
to group by index and name our groups, but thenkey
has to be the value inindex
andvalue
the name of our group. This obv wont work in this case since the index will occur multiple times in different groups. Would be a cool feature though (to be able to do it on your kind ofdict
) :D– user3471881
Nov 22 at 17:13