Takes too long to export data from bigquery into Jupyter notebook
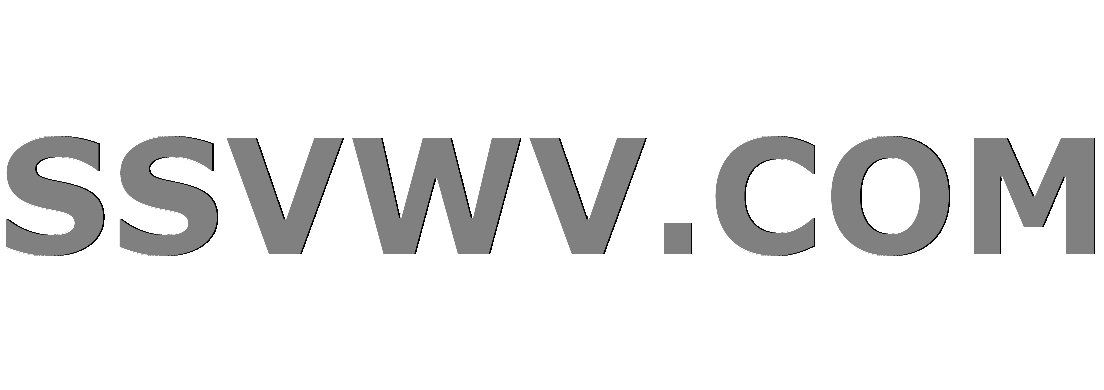
Multi tool use
up vote
4
down vote
favorite
In the Jupyter Notebook, I am trying to import data from BigQuery using an sql-like query on the BigQuery server. I then store the data in a dataframe:
import os
os.environ["GOOGLE_APPLICATION_CREDENTIALS"]="credentials.json"
from google.cloud import bigquery
sql = """
SELECT * FROM dataset.table
"""
client = bigquery.Client()
df_bq = client.query(sql).to_dataframe()
The data has the shape (6000000, 8) and uses about 350MB of memory once stored in the dataframe.
The query sql
, if executed directly in BQ, takes about 2 seconds.
However, it usually takes about 30-40 minutes to execute the code above, and more often than not the code fails to execute raising the following error:
ConnectionError: ('Connection aborted.', OSError("(10060, 'WSAETIMEDOUT')",))
All in all, there could be three reasons for the error:
- It takes the BigQuery server a long time to execute the query
- It takes a long time to transfer data (I don't understand why a 350MB file should take 30min to be sent over the network. I tried using a LAN connection to eliminate server cuts and maximize throughput, which didn't help)
- It takes a long time to set a dataframe with the data from BigQuery
Would be happy to gain any insight into the problem, thanks in advance!
python dataframe

add a comment |
up vote
4
down vote
favorite
In the Jupyter Notebook, I am trying to import data from BigQuery using an sql-like query on the BigQuery server. I then store the data in a dataframe:
import os
os.environ["GOOGLE_APPLICATION_CREDENTIALS"]="credentials.json"
from google.cloud import bigquery
sql = """
SELECT * FROM dataset.table
"""
client = bigquery.Client()
df_bq = client.query(sql).to_dataframe()
The data has the shape (6000000, 8) and uses about 350MB of memory once stored in the dataframe.
The query sql
, if executed directly in BQ, takes about 2 seconds.
However, it usually takes about 30-40 minutes to execute the code above, and more often than not the code fails to execute raising the following error:
ConnectionError: ('Connection aborted.', OSError("(10060, 'WSAETIMEDOUT')",))
All in all, there could be three reasons for the error:
- It takes the BigQuery server a long time to execute the query
- It takes a long time to transfer data (I don't understand why a 350MB file should take 30min to be sent over the network. I tried using a LAN connection to eliminate server cuts and maximize throughput, which didn't help)
- It takes a long time to set a dataframe with the data from BigQuery
Would be happy to gain any insight into the problem, thanks in advance!
python dataframe

40 mins seems a lot indeed...maybe it's the http transport layer. I wonder if you can make two tests: first, what happens if you set a limit to 10000 rows for instance in your query? Is it still slow? Also, can you test first exporting this data to GCS gizzed and then bring it to host to finally read as dataframe, is it still slow?
– Willian Fuks
Nov 22 at 17:59
add a comment |
up vote
4
down vote
favorite
up vote
4
down vote
favorite
In the Jupyter Notebook, I am trying to import data from BigQuery using an sql-like query on the BigQuery server. I then store the data in a dataframe:
import os
os.environ["GOOGLE_APPLICATION_CREDENTIALS"]="credentials.json"
from google.cloud import bigquery
sql = """
SELECT * FROM dataset.table
"""
client = bigquery.Client()
df_bq = client.query(sql).to_dataframe()
The data has the shape (6000000, 8) and uses about 350MB of memory once stored in the dataframe.
The query sql
, if executed directly in BQ, takes about 2 seconds.
However, it usually takes about 30-40 minutes to execute the code above, and more often than not the code fails to execute raising the following error:
ConnectionError: ('Connection aborted.', OSError("(10060, 'WSAETIMEDOUT')",))
All in all, there could be three reasons for the error:
- It takes the BigQuery server a long time to execute the query
- It takes a long time to transfer data (I don't understand why a 350MB file should take 30min to be sent over the network. I tried using a LAN connection to eliminate server cuts and maximize throughput, which didn't help)
- It takes a long time to set a dataframe with the data from BigQuery
Would be happy to gain any insight into the problem, thanks in advance!
python dataframe

In the Jupyter Notebook, I am trying to import data from BigQuery using an sql-like query on the BigQuery server. I then store the data in a dataframe:
import os
os.environ["GOOGLE_APPLICATION_CREDENTIALS"]="credentials.json"
from google.cloud import bigquery
sql = """
SELECT * FROM dataset.table
"""
client = bigquery.Client()
df_bq = client.query(sql).to_dataframe()
The data has the shape (6000000, 8) and uses about 350MB of memory once stored in the dataframe.
The query sql
, if executed directly in BQ, takes about 2 seconds.
However, it usually takes about 30-40 minutes to execute the code above, and more often than not the code fails to execute raising the following error:
ConnectionError: ('Connection aborted.', OSError("(10060, 'WSAETIMEDOUT')",))
All in all, there could be three reasons for the error:
- It takes the BigQuery server a long time to execute the query
- It takes a long time to transfer data (I don't understand why a 350MB file should take 30min to be sent over the network. I tried using a LAN connection to eliminate server cuts and maximize throughput, which didn't help)
- It takes a long time to set a dataframe with the data from BigQuery
Would be happy to gain any insight into the problem, thanks in advance!
python dataframe

python dataframe

edited Nov 22 at 15:48
asked Nov 22 at 14:22
Max Sfnv
211
211
40 mins seems a lot indeed...maybe it's the http transport layer. I wonder if you can make two tests: first, what happens if you set a limit to 10000 rows for instance in your query? Is it still slow? Also, can you test first exporting this data to GCS gizzed and then bring it to host to finally read as dataframe, is it still slow?
– Willian Fuks
Nov 22 at 17:59
add a comment |
40 mins seems a lot indeed...maybe it's the http transport layer. I wonder if you can make two tests: first, what happens if you set a limit to 10000 rows for instance in your query? Is it still slow? Also, can you test first exporting this data to GCS gizzed and then bring it to host to finally read as dataframe, is it still slow?
– Willian Fuks
Nov 22 at 17:59
40 mins seems a lot indeed...maybe it's the http transport layer. I wonder if you can make two tests: first, what happens if you set a limit to 10000 rows for instance in your query? Is it still slow? Also, can you test first exporting this data to GCS gizzed and then bring it to host to finally read as dataframe, is it still slow?
– Willian Fuks
Nov 22 at 17:59
40 mins seems a lot indeed...maybe it's the http transport layer. I wonder if you can make two tests: first, what happens if you set a limit to 10000 rows for instance in your query? Is it still slow? Also, can you test first exporting this data to GCS gizzed and then bring it to host to finally read as dataframe, is it still slow?
– Willian Fuks
Nov 22 at 17:59
add a comment |
active
oldest
votes
active
oldest
votes
active
oldest
votes
active
oldest
votes
active
oldest
votes
Thanks for contributing an answer to Stack Overflow!
- Please be sure to answer the question. Provide details and share your research!
But avoid …
- Asking for help, clarification, or responding to other answers.
- Making statements based on opinion; back them up with references or personal experience.
To learn more, see our tips on writing great answers.
Some of your past answers have not been well-received, and you're in danger of being blocked from answering.
Please pay close attention to the following guidance:
- Please be sure to answer the question. Provide details and share your research!
But avoid …
- Asking for help, clarification, or responding to other answers.
- Making statements based on opinion; back them up with references or personal experience.
To learn more, see our tips on writing great answers.
Sign up or log in
StackExchange.ready(function () {
StackExchange.helpers.onClickDraftSave('#login-link');
});
Sign up using Google
Sign up using Facebook
Sign up using Email and Password
Post as a guest
Required, but never shown
StackExchange.ready(
function () {
StackExchange.openid.initPostLogin('.new-post-login', 'https%3a%2f%2fstackoverflow.com%2fquestions%2f53432996%2ftakes-too-long-to-export-data-from-bigquery-into-jupyter-notebook%23new-answer', 'question_page');
}
);
Post as a guest
Required, but never shown
Sign up or log in
StackExchange.ready(function () {
StackExchange.helpers.onClickDraftSave('#login-link');
});
Sign up using Google
Sign up using Facebook
Sign up using Email and Password
Post as a guest
Required, but never shown
Sign up or log in
StackExchange.ready(function () {
StackExchange.helpers.onClickDraftSave('#login-link');
});
Sign up using Google
Sign up using Facebook
Sign up using Email and Password
Post as a guest
Required, but never shown
Sign up or log in
StackExchange.ready(function () {
StackExchange.helpers.onClickDraftSave('#login-link');
});
Sign up using Google
Sign up using Facebook
Sign up using Email and Password
Sign up using Google
Sign up using Facebook
Sign up using Email and Password
Post as a guest
Required, but never shown
Required, but never shown
Required, but never shown
Required, but never shown
Required, but never shown
Required, but never shown
Required, but never shown
Required, but never shown
Required, but never shown
jCqiVroFEHLQqylpu4f,EveWfrAUDPnKIyLxujRt2hS f 3JGFR,mm58Z2v1oHMBdyLghwU8jYWl,zcDqix cuNjA,d7UQRRhh
40 mins seems a lot indeed...maybe it's the http transport layer. I wonder if you can make two tests: first, what happens if you set a limit to 10000 rows for instance in your query? Is it still slow? Also, can you test first exporting this data to GCS gizzed and then bring it to host to finally read as dataframe, is it still slow?
– Willian Fuks
Nov 22 at 17:59